Latest news about Bitcoin and all cryptocurrencies. Your daily crypto news habit.
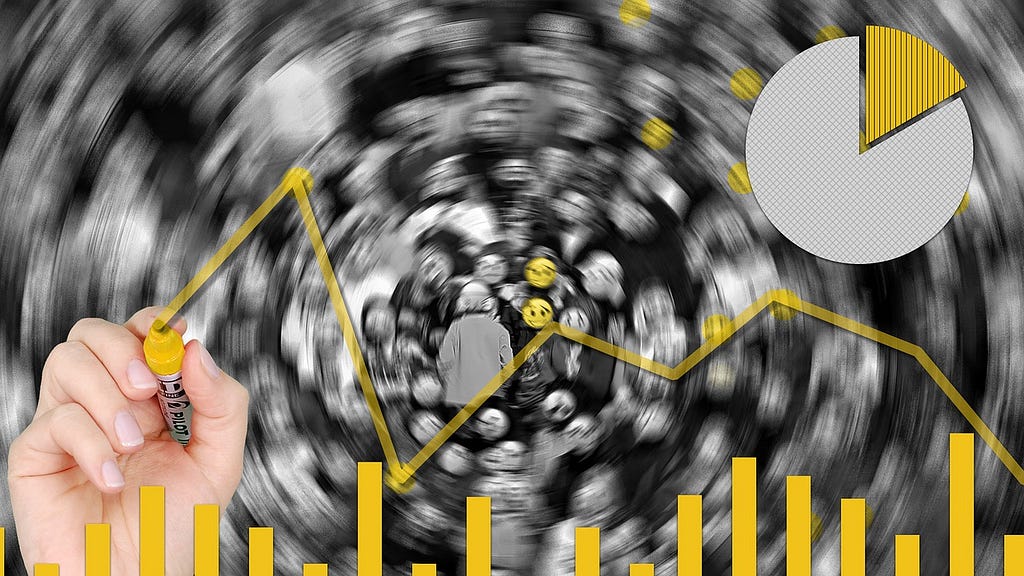
The âera of Big Dataâ
Data availability in the âera of Big Dataâ has increased dramatically. Companies typically accumulate large volumes of complex data, describing an increasing range of business activities. In contrast to old practices, companies now maintain full history of activities and detailed data, to enable advanced modelling and analytical capabilities.
Depending on the industry and the domain, âdata storesâ may contain customer profiles, transactions, customer classifications, customer care activity, sales activities, purchases, productivity metrics, industrial measurements, financial metrics, statistical metadata etc. Modern companies also handle web logs, streams of IoT events, voice and video footage, public domain content and 3rd party data. The opportunities on top of such rich corporate âdata storeâ are unlimited:
with proper processes, analytical models, smart integration and AI-powered experiences, corporations can become smarter and perform better
But, to create business value, you need not only data, but also the right technology, talent and culture: new streams of data create business opportunities but at the same time increase ambiguity, noise and complexity:
âcorporations frequently face a situation described as data overload, perplexing the process of understanding and using the data in the right business contextâ
In plenty of cases, employees and teams are not ready to discover, interpret and use the data in decision-making processes.
To make sense of the data, to systematically leverage valuable insights to better run the business, companies also need the right technologies and a strategic programme, to establish the analytics culture and ensure sufficient readiness to use data and insights in real business problems.
The Technology
The volume and complexity of data requires a stack of advanced technologies, including high-performance data stores, reliable and fast data processing pipelines, powerful analytical models, flexible integration layers and interactive visualization systems.
At the data layer, companies typically use combinations of Data Warehouses, Data Marts, Document Stores and distributed file systems. Some corporations already use Data Lakes to store large volumes of data and content in their original formatâââfurther empowering data-science teams to perform ad-hoc analytical and machine learning projects. Data integration components like ETL, ELT and similar, are also critical here to ensure seamless, reliable flow of high-quality data and content.
At the modelling layer, a range of data mining, statistical modelling and machine learning technologies are typically used to model data, identify trends, patterns and generate insights. Models could be running on a (semi) autonomous modeâââreleased and integrated into production pipelines. For instance, in a CRM context these models make real-time decisions such as request handling, routing and prioritization; or in an Online Media business, machine learning components post-process content for personalization and performance optimization. At this level, data-science teams develop new analytical components, either stand-alone addressing specific business asks, or extensions of existing data processing engines.
At the exploration and presentation layer, Business Intelligence platforms and visualization systems provide access to data models and insights, typically trough reporting, interactive dashboards or OLAP-like data exploration experiences. In the near future, the process of discovering the right insights to support your business needs, will gradually become simplerâââallowing a user to describe a business question and get back the right insights-based answer. As analytical systems become proactive, they will âknowâ who, when and how to notify based on events, trends, outliers and known or newly discovered patterns.
The Culture
To create significant value out of data and analytics, corporations need an additional ingredient: a special mindset, a shared mentality which recognizes data and insights as the catalyst towards more objective and efficient decision-making processes.
In a business environment with a strong analytics culture, data and insights are easily discoverable and accessible. Interesting findings, highlights, statistics and patterns are systematically shared across the right teamsâââin many cases summarized and visualized as âdata storiesâ.
When the âculture of analyticsâ is there, employees ânaturallyâ use data and insights to support their decisions and improve business performance. They define and attach quantifiable success criteria to all those important and measurable activities and decisions. Teams, look for data to test their assumptions and evaluate business outcomes. Product managers optimize product development efforts by combining multiple signals, quantitative and qualitative insights.
Having both the cultural and the technological readiness to consume and interpret data, teams become more effective in discovering business opportunities and forming novel commercial offerings.
The âculture of analyticsâ is also empowering the innovation process: data and insights help teams to better understand, define and communicate the problem space, and accurately structure specific âproblem statementsâ.
Brainstorming and related innovation activities, also benefit from this data-driven mentality and the general availability of rich data and insights.
The Transformation
Establishing a culture of analytics is long process, and it typically requires a special programme. Technology is also needed to make interaction with data easier, while ensuring smooth, controllable and consistent information diffusion throughout the enterprise. The following provides key-factors which can define the extent of success of the transformational initiative.
Data Quality
Data Quality is of critical importance: to establish the data-driven culture, you need to first ensure clean, accurate, reliable and active data, reflecting all major business activities. Teams, need to trust the data and feel confident to use them in important data-driven decisions. Lack of, or limited data quality, can prove to be the single point of failure. Data engineering teams need to make sure that only clean and reliable data become available to the user community.
Read also: Data Quality in the era of A.I.Access to Data and Insights
Companies must empower employees to easily access data and actionable insights. This can be achieved by introducing a âSelf-service Insights storeâ, a layer hiding the complexity associated with the data processing/modelling pipelines, while exposing standardized, high-quality data objects. These data objectsâââmetrics, KPIs, statistics, widgets, visualizationsâââare discoverable, embeddable and well-documented, potentially with references to examples and real business cases. Authorized users, can configure and adjust the data objects, according to the specific business context.
This Insights store empowers teams to better analyse decisions, assumptions or other business activities.Data & technology Awareness
Employees across all levels and functions, need to be aware of the available data, the metrics and KPIs, for both their own business microcosm and the entire corporation. Metrics and KPIs must become part of the common corporate language: must be listed with a solid definition, which is understood and interpreted in the exact same way across the company. This analytics dictionary must be accessible by all authorized users and packaged in the right business context.
Employees and teams must be aware of the âstate of the artâ in reference to technologies such as Artificial Intelligence, Analytics and Visualization.
In an environment with strong Analytics culture, employees are expected to contribute in the data & analytics vision and strategy: to be able to propose new data-driven projects and initiatives, they need to first understand what is feasible and potentially valuableâââbased on the state of the art and the overall business strategy respectively.
Data and Technology awareness can be raised via several educational activities and events. Also, through information sharing activities, such as quick-start sessions socializing data availability, insights and success stories on how data led to smart decisions resulting to quantified financial or other impact. Formal training sessions on data analysis technology is also a great step towards the transition to the analytical mindset.
Insights Diffusion
Insights and interesting data findings need to be shared in the right format, and through the proper channel. Companies need to establish streams of Insights and Data Stories, based on minimalistic, easy-to-consume artefactsâââinstead of multi-page reports, dysfunctional and cross-linked spreadsheets or overloaded dashboards.
âData storiesâ can present specific aspects of the business, the performance of a specific product, the impact of certain decisions, and the results of large-scale online experiments.
Having great insights to share, Data Stories must focus on UX, so each âstoryâ is delivered in a smooth, interactive and engaging way. In an ideal scenario, stories should be personalised, taking into consideration the perspective of each target user (according to role, seniority and line of business). Part of the diffusion mechanism, could be the âconnected buildingâ allowing intelligent insights-sharing scenarios (for instance, via a smart network of screens).
Data-driven (-inspired) Innovation
Having a rich data store can be a great source of inspiration. Companies can organize mini-hackathons, challenging teams to discover known but hidden patterns in the data (artificial or real data sets). In another scenario, teams can be asked to work on known data sets and well-defined predictive or other analytical problems, and compete in increasing accuracy or performance; or ask teams to create innovative visualizations on top of well-understood data sets. At a business level, employees can be asked to ideate on how to make insights actionable; to interpret them in a real or hypothetical business context and propose actions and strategies.
Self-Reference: Insights about Insights
The overall analytical readiness and culture must be measured as well: employees and teams must be able to provide feedback on the analytical capabilities; or submit ideas, needs and requests for new reports, additional data points, or new data models and visualizations. The involved systems should be using telemetry and instrumentation to capture how employees are accessing, sharing and using analytics.
The Insights store need to also expose a set of specialized KPIs reflecting its own performanceâââallowing continuous improvement.The key elements for a successful transition to the analytics culture are data, technology and mindset: you need as much data as possible, powerful analytical tools, creative people and the right culture. This, will gradually enable teams to ânaturallyâ explore, analyse and model data; to inherit a data-driven decisioning approach; to lead strategic analytical initiatives and user-facing, data-driven experiences.
Interested in Artificial Intelligence?
- Artificial Intelligence: the impact on employment and the workforce
- Artificial Intelligence: A non-technical introductionâââdefinition, applications and impact
- Data Quality in the era of A.I.
- Whatâs next on AI, AR, VR, NUI, Robotics, Data & Visualization, Blockchain
- How AI will Impact Transportation
Follow The Innovation Machine for novel ideas and thoughts on innovation!
George Krasadakis | Professional Profile | LinkedIn
Establishing a culture of Analytics was originally published in Hacker Noon on Medium, where people are continuing the conversation by highlighting and responding to this story.
Disclaimer
The views and opinions expressed in this article are solely those of the authors and do not reflect the views of Bitcoin Insider. Every investment and trading move involves risk - this is especially true for cryptocurrencies given their volatility. We strongly advise our readers to conduct their own research when making a decision.