Latest news about Bitcoin and all cryptocurrencies. Your daily crypto news habit.
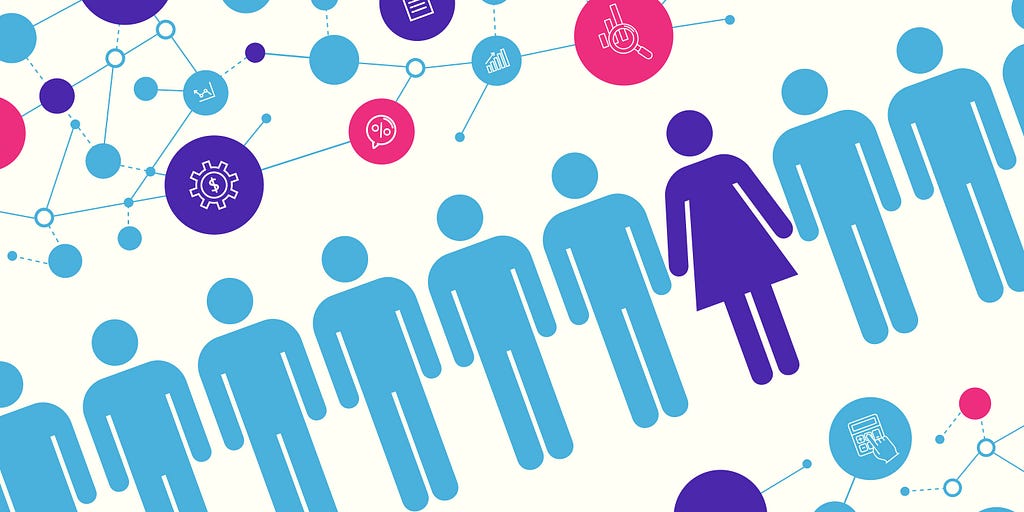
Meet the initiative which helps companies set a better habit to generally consider women for data-intense roles — DSSe ❤. BIG Thank you to Amarita Natt, Sr. Economist at Econ One Research for writing this post and helping us kick off DSSe, an initiative focused on elevating women in Data.
Everyone in Data Science has encountered job listings that ask for the unicorn: someone who is an expert in programming languages and computer science, statistics and econometrics, and business. Oh, and if you can do compelling data visualizations too, that would be great. There are probably people out there who combine all those traits, but they’re few and far between. As the field has evolved, job descriptions have become more specific to one of those fields, and the most successful Data Science teams are exactly that: teams. These teams benefit from specialization and collaboration. An engineer might be in charge of collecting, cleaning, and maintaining the data. A statistician or economist might be running analysis on the data, such as predictive models. There might be a visualization expert figuring out how best to communicate the insights produced by the models, and a business leader guiding the question of interest. At all of those levels are humans, making decisions about the data that is collected, the questions that are asked, and the insights that are delivered.
Data Science teams are successful because each member brings their own training, experience, and perspective to the table. It seems obvious that having a diversity of skillsets allows for more creative problem solving, but often that conclusion is not extrapolated further, to recognize that a diversity of life experience also strengthens a team.
Our world is shaped by algorithms and models, absorbing data and churning out insights at incredible rates. Everything from which television shows are renewed to which groceries are stocked at your local store are determined based on the results of data modeling. Those are relatively innocuous outcomes, but data modeling is also determining how police resources are distributed and whether an individual convicted of a crime is likely to re-offend. In a perfect world, where data had no bias and models didn’t require human input, that might result in optimal outcomes, but we don’t live in that world. Models are only as good as their designers and data doesn’t know what it hasn’t seen.
Even more fundamentally, it is data scientists who are framing the very questions that are considered worth exploring through data.
When you look at it that way, data scientists have a lot of influence, and if data scientists are a homogenous group with similar backgrounds and interests, it narrows the world that we explore and limits the validity of the insights we produce from the data.
That homogeneity isn’t limited to educational or technical backgrounds. According to Burtch Works (via DSSe), 85% of Data Scientists and 74% of Predictive Analysts are male. Considering the fields that make up those professions, the disconnect becomes even more apparent: only 18% of computer science bachelor’s degrees in 2016 were awarded to women, according to the National Center for Education Statistics. Statistics does better, at almost 43% female, and economics (via the National Science Foundation) falls somewhere around 31%, but consider that overall, women earn 57% of all bachelor’s degrees awarded. Given the stark numbers in education, it’s not surprising that women are underrepresented among the Data Science and Predictive Analytics professions. Of course, that lack of representation means that the questions we ask, the variables we use in models, and even our interpretations of those models are all skewed by selection bias within our profession.
Amazon was reminded of this recently: after sinking time and money into an attempt to use AI to improve their hiring practices, all they found was that the model, trained on incredibly biased data composed of their successful past hires, would find ever more subtle ways to filter out women. Amazon had the perspective to understand the limitations of their algorithm (or, more accurately, their dataset), but how many firms apply data science in a hopelessly biased way without ever seeing it? Or what about the case where an algorithm is accurate in its predictions but the application of that knowledge doesn’t consider potentially negative outcomes? Target encountered such a situation several years ago when its data science team was able to accurately predict when a customer was pregnant based on their purchase history, and used that information to market pregnancy- and baby-related items to the customers. What they did not consider is that those marketing circulars go to households, not individuals, and the pregnant person might not want that information shared, as in the case of a teenaged girl who had not yet told her parents of her unplanned pregnancy. As many articles at the time noted, it could have been a disastrous outcome if a woman was hiding her pregnancy due to the risk of violence. In this case, a more diverse team with a wider range of life experience might have noticed the potential danger in the application of their insights.
Without breadth of perspective, you end up with the inevitable failure of imagination. That’s why initiatives like DSSe, working to elevate women in Data Science, matter.
Having more women in Data Science affects everything from the questions we ask to the tools we use to the techniques we explore. By bringing our perspectives, we create better models and answer more meaningful questions.
Intel, for instance, committed to an ambitious plan to increase diversity in its ranks in 2015. Almost three years later, they have improved representation among women and minority groups by 63% and have done so without sacrificing profitability in any way — not a surprise, given Morgan Stanley’s report that highly gender diverse companies can deliver slightly better returns with lower volatility. Data science is still young, and we have the opportunity to shape the field so that it reflects the world it explores. It may skew male now, but with programs like DSSe, mentorship between women in the field, and targeted encouragement of young women considering careers in Data Science, soon Data Science will have the diversity of perspective that can truly elevate the field.
Nominate someone you know today.
Data from a Female Point of View was originally published in Hacker Noon on Medium, where people are continuing the conversation by highlighting and responding to this story.
Disclaimer
The views and opinions expressed in this article are solely those of the authors and do not reflect the views of Bitcoin Insider. Every investment and trading move involves risk - this is especially true for cryptocurrencies given their volatility. We strongly advise our readers to conduct their own research when making a decision.